AI Project Cycle Class 9 Questions and Answers
In this section students will learn some important AI Project Cycle Class 9 Questions and Answers. The Questionnaire is divided in following sections.
AI Project Cycle Class 9 Questions and Answers (MCQs)
AI Project Cycle Class 9 Questions and Answers (Fill in the Blanks)
AI Project Cycle Class 9 Questions and Answers (State true and False)
AI Project Cycle Class 9 Questions and Answers (Short Questions)
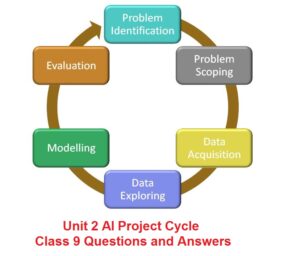
Multiple Choice Questions (MCQs).
1. Which of the following is not a stage in the AI Project Cycle:
a) Problem Scoping
b) Data Acquisition
c) Data Exploration
d) Prototyping
Answer- d) Prototyping
2. While finalising the aim of this system, you scope the problem which you wish to solve with the help of your project. This is called:
a) Data acquisition
b) Problem Scoping
c) Data exploration
d) Modelling
Answer- b) Problem Scoping
3. When you explore the data and try to put it uniformly for a better understanding. It is called:
a) Data acquisition
b) Problem Scoping
c) Data exploration
d) Modelling
Answer- c) Data exploration
4. You go for data acquisition by collecting data from various reliable and authentic sources. This is called:
a) Data acquisition
b) Problem Scoping
c) Data exploration
d) Modelling
Answer- a) Data acquisition
5. ______refers to developing algorithms, also called models which can be trained to get intelligent
a) Data acquisition
b) Problem Scoping
c) Data exploration
d) Modelling
Answer- d) Modelling
AI Project Cycle Class 9 Questions and Answers
6. It shows the components and boundaries of a system and the components of the environment ata specific point in time.
a) System map
b) Sketch graph
c) Decision tree
d) Data features
Answer- a) System map
7. Using this, you visualise the graphical data in a graphical format.
a) System map
b) Sketch graph
c) Decision tree
d) Data features
Answer- b) Sketch graph
8. It is a rule-based Al model which helps the machine in predicting what an element is with the help of various decisions (or rules) fed to it.
a) System map
b) Sketch graph
c) Decision tree
d) Data features
Answer- c) Decision tree
9. In the System map of the water cycle as mentioned in the chapter, If an arrow goes from X to Y with a-(minus) sign, it means that
a) If X increases, Y decreases
b) If X increases, Y increases
c) The direction of relation is opposite
d) It is a bi-directional relationship
Answer- a) If X increases, Y decreases
10. It is the type of Supervised Learning in which labelled data is used to make predictions in a continuous form.
a) Regression
b) Clustering
c) Classification
d) All of the above
Answer- a) Regression
11. A problem is when the output variable is a category, such as “red” or “blue” or “disease” and “no disease”.
a) Regression
b) Classification
c) Clustering
d) All of the above
Answer- b) Classification
AI Project Cycle Class 9 Questions and Answers
State True and False against the statements.
1. The 5Ws Problem Canvas can define Golas of a project. [True]
2. Data features refer to the type of data you want to collect. [False]
3. A decision tree shows the components and boundaries of a system and the components of the environment at a specific point in time. [False]
4. Using the Sketch graph, you visualise the graphical data in a graphical format. [True]
5. A rule-based approach Refers to Al modelling, where the developer pre-defines the relationship or patterns in data. [True]
6. Rule-based approach refers to Al modelling, where the developer does not pr-define the relationship or patterns in data. [False]
7. The basic structure of a Decision Tree starts from the root which the point where the decision tree starts. [True]
8. The stage of testing the models is known as Modelling. [False]
9. Regression is the type of Supervised Learning in which labelled data is used to make predictions in a continuous form. [True]
10. Clustering is the task of partitioning the dataset into groups, called clusters Select the suitable words and fill in the blanks. [True]
AI Project Cycle Class 9 Questions and Answers
Fill in the blanks.
1. The ________ Problem Canvas can define Golas of a project. [4Ws]
2. ______refer to the type of data you want to collect. [Data features]
3. A ____shows the components and boundaries of a system and the components of the environment at a specific point in time. [System map]
4. ___________ enables software to train itself to perform tasks with vast amounts of data. [Deep Learning]
5. A rule-based approach Refers to ________ ,where the developer pre-defines the relationship or patterns in data. [Al modeling]
6. A learning-based approach refers to __________ where the developer does not pre define the relationship or patterns in data. [Evaluation]
7. The basic structure of a Decision Tree starts from the__________, which the point where the decision tree starts. [Root]
8. The stage of testing the models is known as ___________. [Modelling]
9. ___________is the task of partitioning into the groups, called clusters. [Clustering]
10. A_____________ problem is when the output variable is a category, such as “red” or “blue”. [Classification]
AI Project Cycle Class 9 Questions and Answers
Descriptions based questions.
- Which are the elements of 4W canvas?
The 4W canvas is an effective project management tool used to define and communicate project goals and objectives. It contains four elements – What, Why, Whom and When.
What: The “What” element refers to the specific goals or objectives of the project. This component involves outlining exactly what must be achieved or accomplished. If, for instance, your aim is building a website, then its “What” would be creating a user-friendly design.
Why: The “Why” element focuses on the reasons and benefits for undertaking the project, helping to better understand its purpose and significance as well as any problems or opportunities it aims to address; for instance, for website projects this “Why” could include expanding online visibility and reaching more visitors.
Who: This element identifies key stakeholders and individuals involved with a project, including project team members, clients, users and any other relevant parties. A clear understanding of roles and responsibilities among each stakeholder is paramount for efficient project execution.
When: The “When” element refers to the timeline and deadlines of a project. This step includes setting milestones, deliverables and completion dates in order to facilitate planning and scheduling project activities in a timely fashion.
AI Project Cycle Class 9 Questions and Answers
- Define Data acquisition.
Data acquisition refers to the process of collecting information from various sources for use in analysis, decision-making and research purposes. In Simple words, data acquisition involves gathering reliable and pertinent information that provides valuable insights or solves an existing issue or problem. Data can be acquired using various techniques including surveys, interviews, observations experiments or accessing existing datasets.
Acquisition is a critical element of any project or study, serving as the cornerstone for subsequent analysis or modeling. Acquiring data requires identifying specific items needed, selecting sources suitable for them, and employing collection techniques – with emphasis placed upon maintaining data quality while upholding integrity standards and adhering to ethical considerations.
AI Project Cycle Class 9 Questions and Answers
- Define Problem Scoping.
Problem scoping refers to the process of clearly defining and comprehending a specific issue or challenge that a project aims to solve, by setting boundaries, identifying key objectives, and determining its scope. Problem scoping helps in providing clarity to projects by aligning stakeholders’ expectations with expectations of all involved and providing clear direction for project teams.
Problem scoping requires conducting research, gathering information, and analyzing the current situation to gain a fuller understanding of the issue at hand. This may include identifying root causes and potential constraints or limitations as well as any relevant requirements or constraints that must be considered during problem scoping.
Problem scoping results in a well-articulated problem statement that captures its essence, context and impact on an organisation or project. This statement acts as a roadmap for subsequent decision making, resource allocation and solution creation processes.
Problem scoping is an essential step in project management and problem-solving as it sets the groundwork for its success. By creating a shared understanding of the issue and outlining a clear roadmap to finding an optimal solution, problem scoping ensures all parties involved understand it fully.
AI Project Cycle Class 9 Questions and Answers
- Define Data exploration.
Data exploration refers to the practice of investigating and analyzing a dataset in order to gain a greater understanding of its characteristics, patterns, and relationships. It involves investigating data from different angles before exploring its structure in search of insights or trends that could provide direction for decision-making or further examination.
Data exploration involves employing various tools and techniques to examine a dataset, such as summary statistics, data visualization, and exploratory data analysis methods. The goal is to unearth hidden patterns, outliers, correlations and anomalies within the data.
Data exploration enables analysts and researchers to uncover critical features, relationships, or variables that can significantly enhance analysis or decision-making processes. By exploring data, analysts and researchers can quickly identify data quality issues such as missing values and potential biases as well as make informed decisions regarding preprocessing, feature engineering and selecting suitable modeling techniques.
AI Project Cycle Class 9 Questions and Answers
- Explain Modelling.
Modelling in data analysis and machine learning refers to the process of creating algorithms or mathematical representations capable of capturing patterns, relationships, or behaviors within a dataset. Models built using available data may then be used for prediction, classification or insight generation purposes.
Modeling typically comprises these steps:
Data Prep: Data preparation involves preprocessing and transforming raw data in order to make it suitable for modeling. This may involve tasks like data cleaning, feature selection, normalization or even encoding categorical variables.
Model Selection: Selecting an optimal model or algorithm that suits a given problem and dataset. This decision depends on various factors such as data type, problem at hand (regression, classification or clustering) and desired outcomes.
Training the Model: At this step, data collected during preparation are used to train a model selected by you. As it learns from this training data, your model adapts its parameters or internal structure so as to minimize errors or maximize performance on its training data set.
Model Evaluation: Evaluating the performance of a trained model through evaluation metrics and techniques to establish its accuracy, precision, recall or other relevant measures is vital to ensuring its effectiveness as well as to identify any issues or limitations within its structure.
Model Deployment and Prediction: Once trained and evaluated, models can be deployed to make predictions or generate insights on unknown data sets. The model utilizes its knowledge of patterns or relationships learned during training to make classifications and predictions about unknown points of data.
AI Project Cycle Class 9 Questions and Answers
- What is a Decision tree? Define its structure.
Decision trees are an extremely effective machine learning algorithm used for both classification and regression tasks. Their flowchart-like structure demonstrates decisions based on certain features or attributes; each internal node represents one attribute or feature while every branch represents possible values or outcomes of that feature, with leaf nodes representing the ultimate decision or prediction.
A decision tree consists of three primary components. These components include the root node (which represents all data or features leading to subsequent decisions) as the starting point, branches connecting this node with other internal nodes, and its end nodes as decisions made from it.
Internal Nodes: Internal nodes represent features or attributes that contribute to decision-making processes, with each node having an associated decision rule or condition based on the attribute’s value and providing guidance as to which path should be followed through the decision tree based on this attribute.
Leaf Nodes (Target Nodes): Leaf nodes, also referred to as terminal nodes, represent the final results or predictions of a decision tree. They show class labels or numerical values associated with specific paths in the tree and each leaf node contains a prediction or decision based on all attribute values from root to each leaf in question.
Decision trees provide an easy and understandable representation of decisions based on given features, by traversing from its root node to its appropriate leaf nodes, the algorithm can make predictions or classifications for new instances.
AI Project Cycle Class 9 Questions and Answers
- What is the difference between clustering and regression?
Here are the main differences between clustering and regression:
Points of Differentiation | Clustering | Regression |
Goal | In clustering, the aim is to group similar data points together according to their intrinsic characteristics or similarities. | Regression aims to estimate or predict a continuous numerical value based on input variables. |
Task Type | Clustering is an unsupervised learning task, meaning it does not require labeled data or specific target values, with its focus being to find patterns or structures in data.
|
Regression is a supervised learning task, meaning it requires labeled training data with known output values to explore the relationship between input variables and target variables. |
Output | Its Clustering is used to organize data points based on their similarities. It does not assign specific numerical values or labels to its clusters. | Regression produces an estimated numerical value or continuous variable from its input features, with an aim to identify a line or curve which best represents their relationship. |
Interpretation | Clustering results are frequently used to uncover patterns, similarities or groupings within data. Clustering helps in identifying natural clusters or segments within datasets. | Regression models provide interpretable coefficients or parameters that measure the impact or influence of each input variable on predicted output, providing insight into their relationship. |
Data Format | Clumping can work with various forms of data, from numerical to categorical or mixed types, in order to find similarities and patterns regardless of data type. | Regression typically deals with numerical data for both input features and target variables, assuming either a linear or nonlinear relationship between these variables. |