Unit I- Introduction to Artificial Intelligence (817) Class 9
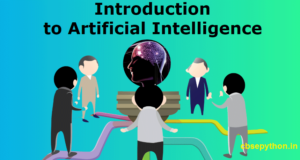
Introduction to Artificial Intelligence Fill in the Blanks :
- _______________test checks the machine’s ability to exhibit intelligent behaviour. [Turing test]
- __________________year is known as AI winter.[1974]
- Microsoft introduced the ________ software in 2014.[Cortana]
- ______________are based on data that Google collects about you.[Predictive searches]
- ______________is the type of AI that can understand or learn any intellectual task that a human being can.[AGI (Artificial General Intelligence)]
- A ___________is a computer program designed to simulate conversation with human users, especially over the Internet.[Chatbot]
- A__________ allows homeowners to control appliances, thermostats, lights, and other devices remotely using a smartphone or tablet through an internet connection.[Smart home]
- An Al-powered_____________ accepts voice commands to create to-do lists, order items online, set reminders, and answer questions (via internet searches).[Personal assistant]
- _____________is a subfield of machine learning.[Deep learning]
- The program called ______________defeated the Go champion.[AlphaGo]
- ___________refers to data that is so large, fast or complex that it is difficult or impossible to process using traditional methods. [Big Data]
- Al needs data to recognize___________. [Patterns]
- ___________use big data to anticipate customer demand. [Netflix]
- Data is the ___________to drive our digital economies. [New oil]
- ___________ depends heavily on Big Data for success. [ΑΙ]
- ___________lets users point a smartphone camera at a sign in another language and almost immediately obtain a translation of the sign in their preferred language.’ [Google Translate]
- _________ is to figure out how all the words in our sentence relate to each other. [Dependency parsing]
- IBM apply Computer vision to diagnose ___________. [Skin cancer]
- ___________is the process of finding the root word of a given the word. [Lemmatization]
Introduction to Artificial Intelligence true or false statements.
- Artificial intelligence today is rightly known as narrow AI.[True]
- AGI systems are used to assist doctors.[False]
- Banks use Al is by sending mobile alerts to help prevent against fraud.[True]
- Ahuman-machine interface is also known as a man-machine interface (MMI).[False]
- To expertise in AI, programmers should not have a curious and creative mindset[False]
- A smart home allows homeowners to control appliances, thermostats, lights, and other devices remotely using a smartphone or tablet through an internet connection.[True]
- Smart Governance is a feature of the Smart City.[True]
- Engineers do not need to learn programming languages such as Python, C++, Rand Java.[False]
- Deep Learning is a subfield of Algorithm Bias.[False]
- Robotic science is used for multiple functions from space exploration, healthcare, security to many other scientific fields.[True]
- Neural networks do not need any data. [False]
- Al is all about algorithms and data. [True]
- By 2022, every person on earth will generate 11MB of data every second. [False]
- Computer Vision (CV) is more dependent on Deep learning. [True]
- Augmented Reality lets you combine computer-generated atmosphere into a real-world atmosphere. [True]
- Any data that can be stored, accessed and processed in the form of fixed-format is termed as a ‘structured’ data. [False]
- In 2011, Facebook began the use of facial recognition. [True]
- Dependency parsing is the process of finding the root word of a given the word. [True]
- Deep learning relies on neural networks. [True]
- Stop words are words such as “the”, “for”, “is” etc., which do not add any value to the meaning of a sentence. [True]
Introduction to Artificial Intelligence Short Answer type Questions:
1. Why is the Turing test performed?
The Turing test is performed to evaluate a machine’s ability to exhibit intelligent behavior indistinguishable from that of a human. It was proposed by Alan Turing in 1950 as a way to determine if a machine can exhibit human-like intelligence.
2. What are the types of AI?
There are various types of AI, including:
a) Narrow or Weak AI: This type of AI is designed to perform specific tasks or functions and is focused on a limited area. Examples include voice assistants like Siri or Alexa.
b) General AI: General AI refers to AI systems that possess human-level intelligence and can understand, learn, and apply knowledge across various domains. However, true general AI does not yet exist and remains a goal for future development.
c) Superintelligent AI: This refers to AI systems that surpass human intelligence and possess exceptional cognitive capabilities. Superintelligent AI is hypothetical and represents a level of AI that surpasses human intellectual capacity.
3. Write the name of applications of Al in daily life?
Some applications of AI in daily life include:
a) Virtual assistants: Voice-activated virtual assistants like Siri, Alexa, or Google Assistant help with tasks such as setting reminders, answering questions, and controlling smart home devices.
b) Recommendation systems: AI-powered recommendation systems suggest products, movies, music, or articles based on user preferences and behavior.
c) Image and speech recognition: AI is used in applications like facial recognition, object detection, and speech-to-text conversion, improving the accuracy and efficiency of these technologies.
d) Autonomous vehicles: AI plays a crucial role in self-driving cars, enabling them to perceive the environment, make decisions, and navigate safely.
e) Personalized healthcare: AI is used in areas like medical imaging analysis, drug discovery, and personalized treatment recommendations.
4. What are simple and smart chatbots?
Simple chatbots are basic AI systems that follow predefined rules and provide programmed responses based on keywords or patterns. They have limited capabilities and cannot understand complex queries beyond their programmed responses.
Smart chatbots, on the other hand, utilize advanced AI techniques such as natural language processing and machine learning. They can understand and interpret user queries, learn from interactions, and provide more intelligent and contextually relevant responses.
5. Define smart city?
A smart city is a concept that describes the integration of technology and data-driven solutions to improve the quality of life for its residents. It involves the use of various technologies, including AI, to enhance urban infrastructure, services, and sustainability. Smart cities aim to optimize resource usage, improve transportation, enhance public safety, promote efficient governance, and provide better overall living conditions for citizens.
6. What are the main features of a Smart school?
The main features of a smart school may include:
a) Digital classrooms: Smart schools incorporate technology such as interactive whiteboards, tablets, and educational software to enhance the learning experience.
b) Personalized learning: AI-powered educational platforms can adapt to individual student needs, providing customized learning paths and personalized feedback.
c) Smart infrastructure: Schools can implement automated systems for tasks like attendance tracking, security, and energy management.
d) Data-driven decision making: Smart schools use data analytics to track student performance, identify areas for improvement, and optimize teaching strategies.
e) Collaboration tools: AI-based collaboration tools enable students and teachers to work together remotely, share resources, and engage in interactive learning experiences.
7. What is human-machine interaction? Write about HYMI interface with some examples.
Human-machine interaction (HMI) refers to the communication and interaction between humans and machines or computers. It involves the design and development of interfaces that allow users to interact with machines effectively and intuitively.
An example of HMI is a touch screen interface on a smartphone, where users can interact with the device by tapping, swiping, or typing. Another example is a voice-controlled virtual assistant like Siri or Alexa, where users can give commands or ask questions using natural language.
8.Name Al career opportunities.
AI career opportunities include:
a) Machine learning engineer: They develop and implement machine learning models and algorithms for various applications.
b) Data scientist: They analyze and interpret complex data to derive insights and build predictive models using machine learning techniques.
c) AI research scientist: They work on cutting-edge research in AI, developing new algorithms and advancing the field.
d) AI ethics specialist: They focus on the ethical implications of AI technologies and ensure their responsible and fair use.
e) AI software developer: They design and develop software applications that utilize AI techniques and algorithms.
f) Robotics engineer: They work on designing, building, and programming robots with AI capabilities.
g) AI consultant: They provide expertise and guidance on implementing AI solutions in various industries.
9.Which skills are required to become a data scientist?
To become a data scientist, some essential skills include:
a) Strong programming skills: Proficiency in languages like Python or R is crucial for data manipulation, analysis, and building machine learning models.
b) Statistical knowledge: Understanding statistical concepts and techniques is important for analyzing data and drawing meaningful conclusions.
c) Machine learning expertise: Knowledge of machine learning algorithms, techniques, and frameworks is essential for building predictive models.
d) Data visualization: The ability to effectively visualize and communicate data insights using tools like Matplotlib or Tableau.
e) Domain knowledge: Familiarity with the specific domain or industry in which the data scientist operates allows for better context and more relevant analysis.
f) Problem-solving skills: Data scientists need to approach complex problems with a logical and analytical mindset.
10.What are machine learning engineers expecting to know?
Machine learning engineers are expected to have a deep understanding of machine learning algorithms, techniques, and frameworks. They should be proficient in programming languages like Python or R and have experience in data preprocessing, feature engineering, and model evaluation. Knowledge of statistical concepts and data visualization is also important. Additionally, machine learning engineers should be familiar with software engineering principles, version control systems, and deployment techniques to operationalize machine learning models efficiently.
Questions from AI Domains
- Define big data? Why does AI need data?
Big data refers to a vast volume of data that is characterized by its high volume, velocity, and variety. It includes both structured and unstructured data from various sources. The key characteristics of big data are commonly referred to as the three Vs: volume (large amount of data), velocity (speed at which data is generated and processed), and variety (different types of data).
AI, or Artificial Intelligence, relies on data to learn, make decisions, and perform tasks. Data serves as the fuel for AI systems, enabling them to train and improve their performance. The more data AI has access to, the better it can understand patterns, make accurate predictions, and provide meaningful insights. Big data provides a rich and diverse collection of information that helps AI algorithms to train and generalize effectively.
- What are the sources of big data?
Big data can be sourced from various places, including:
- Social media platforms: Data generated from social media platforms like Facebook, Twitter, Instagram, and LinkedIn, including posts, comments, likes, and shares.
- Internet of Things (IoT) devices: Data generated by sensors and devices connected to the internet, such as smartwatches, thermostats, cameras, and industrial sensors.
- Online transactions: Data collected from e-commerce websites, online banking, and other digital transactions.
- Scientific research: Data collected from experiments, simulations, and observations in scientific fields such as astronomy, genomics, and climate science.
- Financial records: Data from banking transactions, stock market activities, credit card transactions, and financial statements.
- Government databases: Data collected by government agencies, such as census data, healthcare records, and public services data.
- Web and search data: Data obtained from web pages, search engines, and web logs.
These are just a few examples, and big data can come from a wide range of sources depending on the industry and application.
- Explain the difference between structured, unstructured, and semi-structured big data?
Structured data refers to well-organized and easily searchable data that is typically stored in databases with a predefined schema. It has a fixed format and is organized into rows and columns. For example, data in a relational database, such as customer information or sales records, is structured data. It can be easily queried and analyzed using traditional database management systems.
Unstructured data, on the other hand, lacks a predefined structure and does not fit neatly into traditional databases. It includes data like text documents, emails, social media posts, images, videos, audio files, and sensor data. Unstructured data does not have a fixed format or organization, making it challenging to process and analyze using traditional methods. However, it contains valuable insights that can be extracted using advanced techniques like natural language processing, computer vision, and machine learning.
Semi-structured data falls between structured and unstructured data. It has some structure but is not as rigid as structured data. Semi-structured data contains tags, labels, or other markers that provide a partial organization or hierarchy. Examples include data in XML (eXtensible Markup Language) or JSON (JavaScript Object Notation) formats. Semi-structured data allows for more flexibility and can be processed using techniques designed for structured and unstructured data.
- Define computer vision?
Computer vision is a field of AI that focuses on enabling computers to understand and interpret visual information from images or videos. It involves developing algorithms and techniques that allow machines to analyze, process, and extract meaningful insights from visual data. Computer vision aims to replicate human vision capabilities by enabling machines to perceive and comprehend the visual world.
Computer vision algorithms can perform tasks such as object detection and recognition, image classification, image segmentation, facial recognition, gesture recognition, and video analysis. These algorithms use image processing techniques, machine learning, and deep learning models to analyze and interpret visual data.
- What is the limitation of computer vision?
While computer vision has made significant advancements, it still faces some limitations:
- Ambiguity and complexity: Computer vision algorithms can struggle with interpreting complex or ambiguous visual scenes. Factors like occlusion (objects being partially hidden), poor image quality, variations in lighting conditions, or viewpoint changes can impact the accuracy of computer vision systems.
- Context understanding: Understanding the context of visual data, including background information and the relationships between objects, is challenging for computer vision algorithms. Contextual understanding is crucial for accurate interpretation and decision-making.
- Lack of common sense: Computer vision algorithms may lack common sense reasoning, which humans possess. They may struggle with understanding abstract concepts, subtle cues, or implicit information that humans can easily comprehend.
- Domain-specific knowledge: Computer vision algorithms trained on specific datasets may struggle when applied to new or unfamiliar domains. They require extensive training on diverse and representative data to generalize effectively.
- Define NLP?
NLP stands for Natural Language Processing. It is a branch of AI that deals with the interaction between computers and human language. NLP enables machines to understand, interpret, and generate human language, including speech and text. The goal of NLP is to bridge the gap between human language and machine understanding.
NLP involves various tasks such as text tokenization (breaking text into smaller units like words or sentences), part-of-speech tagging (labeling words with their grammatical categories), syntactic and semantic analysis (parsing the structure and meaning of sentences), language modeling (predicting the next word in a sentence), machine translation (converting text from one language to another), sentiment analysis (determining the sentiment expressed in text), and information extraction (identifying and extracting specific information from text).
- Explain the working of NLP?
The working of NLP typically involves the following steps:
- Text preprocessing: This step involves cleaning and preparing the text data for analysis. It may include tasks like removing punctuation, converting text to lowercase, handling contractions, removing stop words (common words like “and,” “the,” etc.), and applying stemming or lemmatization.
- Tokenization: Tokenization breaks the text into smaller units, such as words, sentences, or subwords. This step creates a structured representation of the text for further analysis.
- Syntactic and semantic analysis: NLP algorithms analyze the structure and meaning of sentences. This includes tasks like part-of-speech tagging, syntactic parsing to determine the grammatical structure, and semantic analysis to understand the meaning and relationships between words.
- Machine learning and deep learning: NLP often leverages machine learning and deep learning techniques to train models on labeled datasets. These models can then be used to perform tasks such as sentiment analysis, named entity recognition, machine translation, and question answering.
- Evaluation and refinement: NLP models are evaluated based on their performance metrics, such as accuracy, precision, recall, or F1 score. The models are refined and fine-tuned using iterative processes to improve their performance.
- What are the uses of NLP?
NLP has numerous applications across various industries and domains:
- Sentiment analysis: NLP can determine the sentiment expressed in text, such as positive, negative, or neutral sentiments. It is used in social media monitoring, customer feedback analysis, and brand reputation management.
- Machine translation: NLP enables the translation of text from one language to another. It is used in language translation services, cross-lingual information retrieval, and localization of software and websites.
- Chatbots and virtual assistants: NLP powers chatbots and virtual assistants, allowing them to understand and respond to natural language queries. They are used in customer support, information retrieval, and voice-controlled smart devices.
- Information extraction: NLP techniques can extract specific information from unstructured text, such as extracting names, dates, locations, or events from news articles or documents.
- Text summarization: NLP algorithms can automatically generate summaries of long texts, enabling efficient information extraction from large volumes of text.
- Voice recognition and speech synthesis: NLP enables systems to recognize and transcribe speech into text and convert text into spoken words. It is used in voice assistants, dictation software, and speech-to-text applications.
These are just a few examples, and NLP has a wide range of applications in various fields.
- Define text parsing?
Text parsing is the process of analyzing and extracting meaningful information from a text by breaking it down into its constituent parts, such as words, phrases, or sentences. It involves analyzing the grammatical structure and syntax of the text to understand its meaning.
Text parsing can involve several subtasks, including:
- Lexical analysis: This involves breaking down the text into individual tokens, such as words or subwords, by identifying word boundaries and removing punctuation marks.
- Syntactic parsing: This task aims to determine the grammatical structure of the text, including the relationships between words and phrases. It involves identifying the subject, object, verb, modifiers, and other grammatical components.
- Semantic analysis: This step focuses on understanding the meaning of the text by analyzing the relationships between words and phrases. It involves determining the entities, actions, or events described in the text.
Text parsing is essential for various NLP tasks, such as machine translation, information extraction, sentiment analysis, and question answering.
- What is Lemmatization? Explain with an example.
Lemmatization is the process of reducing words to their base or dictionary form, called the lemma. The goal is to group together different inflected forms of a word, such as plurals, verb conjugations, or different tenses, under a single lemma. Lemmatization helps in standardizing words for analysis and improving information retrieval or text understanding tasks.
For example, consider the words “running,” “runs,” and “ran.” The lemma for all these words is “run.” Lemmatization reduces these inflected forms to their base form or lemma, which helps in treating them as the same word during analysis.
Similarly, for the word “better,” the lemma would be “good,” as “better” is the comparative form of the adjective “good.” Lemmatization allows for consistent representation and analysis of words with similar meanings, even if they have different forms.